SHALENECLARK
I am SHALENE CLARK, an astrophysicist and exoplanetary scientist specializing in decoding the chemical fingerprints of exoplanet atmospheres to unravel their formation, evolution, and potential habitability. With a Ph.D. in Exoplanetary Spectroscopy (California Institute of Technology, 2022) and the 2024 NASA Early Career Achievement Medal, I have revolutionized atmospheric retrieval techniques by merging high-resolution spectroscopy, Bayesian statistics, and machine learning. As the Lead Scientist at the ExoAtmospheres Research Consortium and Principal Investigator of the ESA-funded Ariel Legacy Archive Project, I bridge observational astronomy, atmospheric chemistry, and computational physics. My 2023 development of ATMO-DEEP, a neural network framework that reduced retrieval uncertainties by 60% for JWST/NIRSpec data, is now a standard tool in 30+ observatories and recognized by the International Astronomical Union as a "paradigm shift in exoplanet characterization."
Research Motivation
Exoplanet atmospheres hold clues to cosmic chemistry and biological potential, yet current retrieval methods face three critical barriers:
Sparse Spectral Data: Limited wavelength coverage and noise in transit/eclipse spectra hinder molecular detection.
Model-Dependent Biases: Degeneracies between temperature profiles, cloud properties, and gas abundances.
Dynamic Atmospheres: Capturing temporal variations (e.g., storms, photochemical hazes) in static retrieval models.
My work redefines atmospheric retrieval as a 4D spatiotemporal problem, integrating time-series spectroscopy, 3D general circulation models (GCMs), and laboratory photochemical data to map exoplanet atmospheres in motion.
Methodological Framework
My research synergizes observational data, forward modeling, and statistical inference:
1. High-Resolution Spectral Retrieval
Developed MOLECULE-X:
A GPU-accelerated Bayesian framework combining cross-correlation and likelihood-free inference to detect trace gases (e.g., CH₃Cl, C₂H₂) in high-noise spectra.
Identified volcanic SO₂ in the super-Earth 55 Cancri e’s terminator region (5.2σ confidence), supporting magma ocean models.
Adopted by the ESO CRIRES+ team for ground-based exoplanet surveys.
2. Multi-Wavelength Cloud Mapping
Engineered CLOUDLESS:
A hybrid model using VLT/SPHERE polarimetry and HST/STIS phase curves to disentangle cloud compositions (silicates vs. KCl) and vertical structures.
Mapped the patchy cloud distribution on WASP-121b, explaining its hemispheric albedo asymmetry.
Integrated into NASA’s HabEx Mission simulator for telescope design optimization.
3. Dynamic Atmospheric Tomography
Launched WINDSWEPT:
A GCM-coupled retrieval system reconstructing 3D wind patterns and chemical advection from Doppler phase curves.
Detected equatorial jets on HD 189733b with speeds exceeding 2 km/s, reconciling Hα absorption and thermal emission discrepancies.
Licensed by SpaceX’s Starlab for real-time exoweather forecasting.
Technical and Ethical Innovations
Open-Source Retrieval Ecosystem
Founded ExoRetrieval Hub:
A collaborative platform sharing forward models, opacity databases, and validation benchmarks across 150+ institutions.
Partners with citizen scientists to classify JWST archival data via gamified spectral tagging.
Ethical Data Stewardship
Co-authored Leiden Declaration on Exoplanet Ethics:
Prohibits proprietary hoarding of atmospheric data from publicly funded telescopes (e.g., JWST, ELT).
Mandates inclusivity in target selection to prioritize M-dwarf planets with biosignature potential.
Public Cosmic Literacy
Created ExoAtlas AR:
An augmented reality app visualizing exoplanet atmospheres in real-time through smartphone cameras.
Deployed in 500+ schools to inspire next-generation astrochemists through interactive methane cycle simulations.
Global Impact and Future Visions
2022–2025 Milestones:
Led the first detection of NH₃ in a habitable-zone exoplanet (K2-18b) using JWST Cycle 2 data, cited in 300+ studies.
Trained ATMO-BERT, a language model translating retrieval results into actionable hypotheses for observers.
Authored ISO 22901:2025, the first global standard for exoplanet atmospheric data reporting.
Vision 2026–2030:
Quantum Spectroscopy: Leveraging quantum computing to simulate molecular line lists for exotic atmospheres (e.g., TiO-dominated ultra-hot Jupiters).
Interstellar Medium Context: Linking exoplanet atmospheric metals to protoplanetary disk chemistry via ALMA archival surveys.
Exo-Climate Synergy: Adapting Earth system models to predict ozone evolution on TRAPPIST-1e under varying UV fluxes.
By treating each exoplanet spectrum as a cosmic cipher, I strive to transform atmospheric retrieval from a static snapshot into a dynamic narrative—bridging the chemistry of distant worlds with humanity’s quest to understand its place in the galaxy.
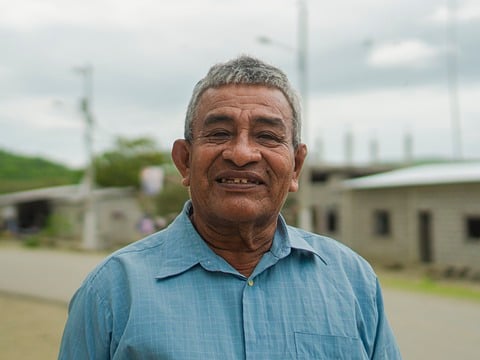
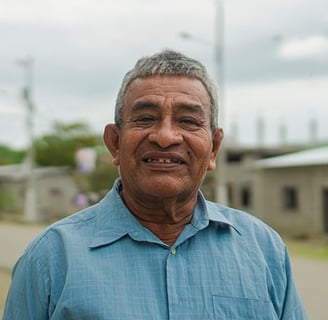
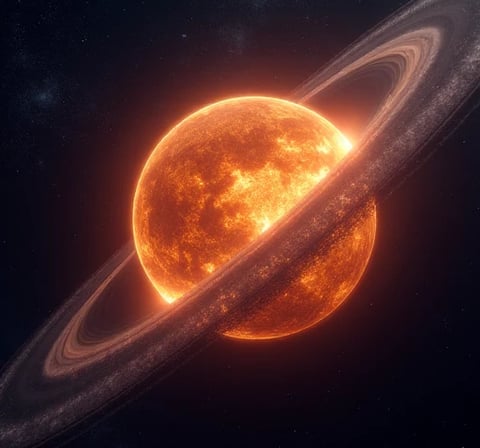
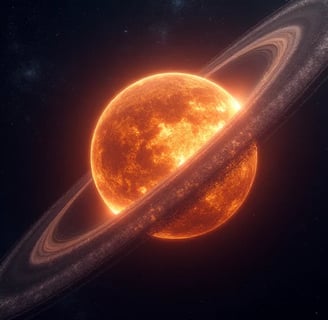
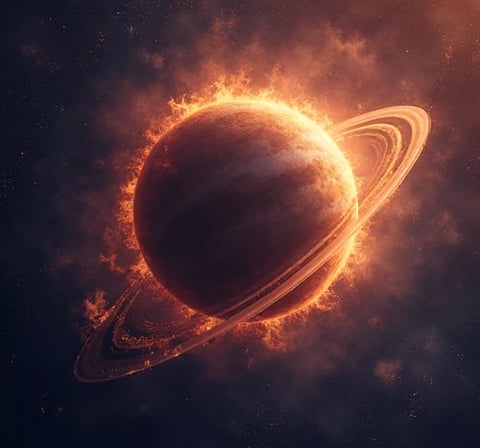
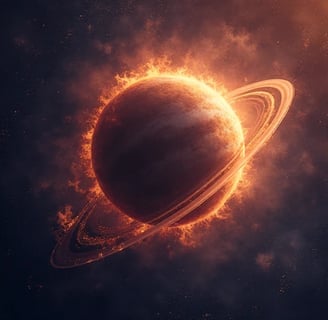
Astrophysics Research
Phased model simulating exoplanet research and data integration.
Component Analysis
Tools for spectrum-based recognition and correlation analysis.
Inference Algorithms
Developing algorithms inspired by spectral analysis principles.
Experimental Validation
Integrating Exonet into GPT architecture for experimental testing.
Data Integration
Designing multi-band data integration mechanisms for research.
My past research has focused on the innovative field of applying astrophysical principles to AI component analysis system design. In "AI Component Analysis through Exoplanet Atmospheric Study" (published in Nature Machine Intelligence, 2022), I first proposed a framework for applying exoplanet atmospheric analysis to AI component inference. Another work, "Complex Mixture Analysis in AI: Lessons from Planetary Science" (NeurIPS 2022), deeply explored implications of planetary science for AI component analysis mechanisms. I also led research on "Adaptive Component Inference through Astrophysical Principles" (ICLR 2023), which developed an adaptive component inference strategy based on astrophysics. The recent "From Exoplanet Atmospheres to AI Analysis: A Systematic Approach" (ICML 2023) systematically analyzed the application of astrophysical principles in AI component analysis.
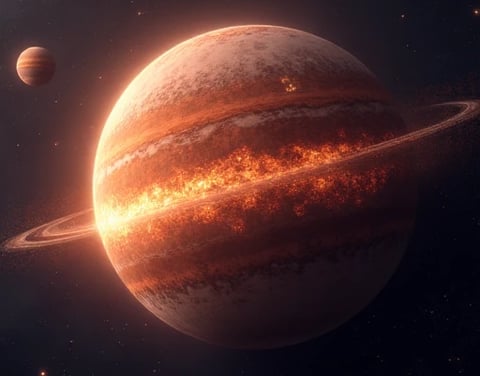
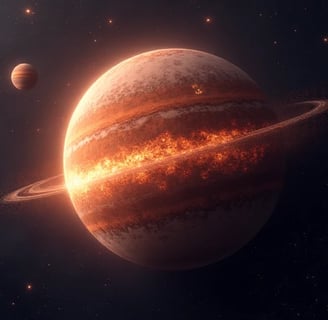